Basic Techniques and Keywords Used in Data Analysis
Data analysis techniques play a critical role in the decision-making process in today’s competitive business world. In this article, we provide a comprehensive guide to the basic techniques and keywords of data analysis. You will learn a wide range of information from data collection methods to machine learning. Let’s get started!
Data Collection Methods
The first step in the data analysis process is to determine the right data collection methods. This stage directly affects the reliability and accuracy of the analysis. Basic data collection methods are as follows:
- Surveys: Surveys that include a variety of questions are ideal for collecting data from large audiences.
- Observation: Collecting data through direct observation is used especially in behavioral analyses.
- Experiments: Experiments conducted under controlled conditions are used to determine cause-effect relationships.
- Existing Records: Data obtained from existing databases of institutions and organizations.
Data collection methods directly affect the quality of the data and the reliability of the analysis results. Therefore, it is of great importance that the method used is suitable for the purpose.
Data Cleaning and Preprocessing Techniques
Collected data may not always be clean and ready for use. Therefore, data cleaning and preprocessing techniques are of great importance. This process makes the data suitable for analysis.
- Missing Data Management: Missing data can reduce the meaningfulness of the data. Therefore, filling, removal or estimation methods are used.
- Noise Reduction: Cleaning the meaningless and random information in the data.
- Data Transformation: Standardizing the data in a certain format.
- Feature Engineering: Deriving new features to make the data more meaningful.
These techniques increase the quality of the data and make the analysis results more reliable.
Descriptive Statistics
Descriptive statistics are basic statistical techniques used to summarize and understand the data set. These techniques reveal the general characteristics of the data.
- Average: Shows the general trend of the data. The arithmetic mean is the most commonly used.
- Median: Represents the middle value of the data.
- Mode: The most frequently occurring value in the data.
- Standard Deviation: Measures the spread and distribution of the data.
- Quartiles: Dividing the data into specific percentiles.
These statistics provide basic information to understand the structure of the data and use in analysis.
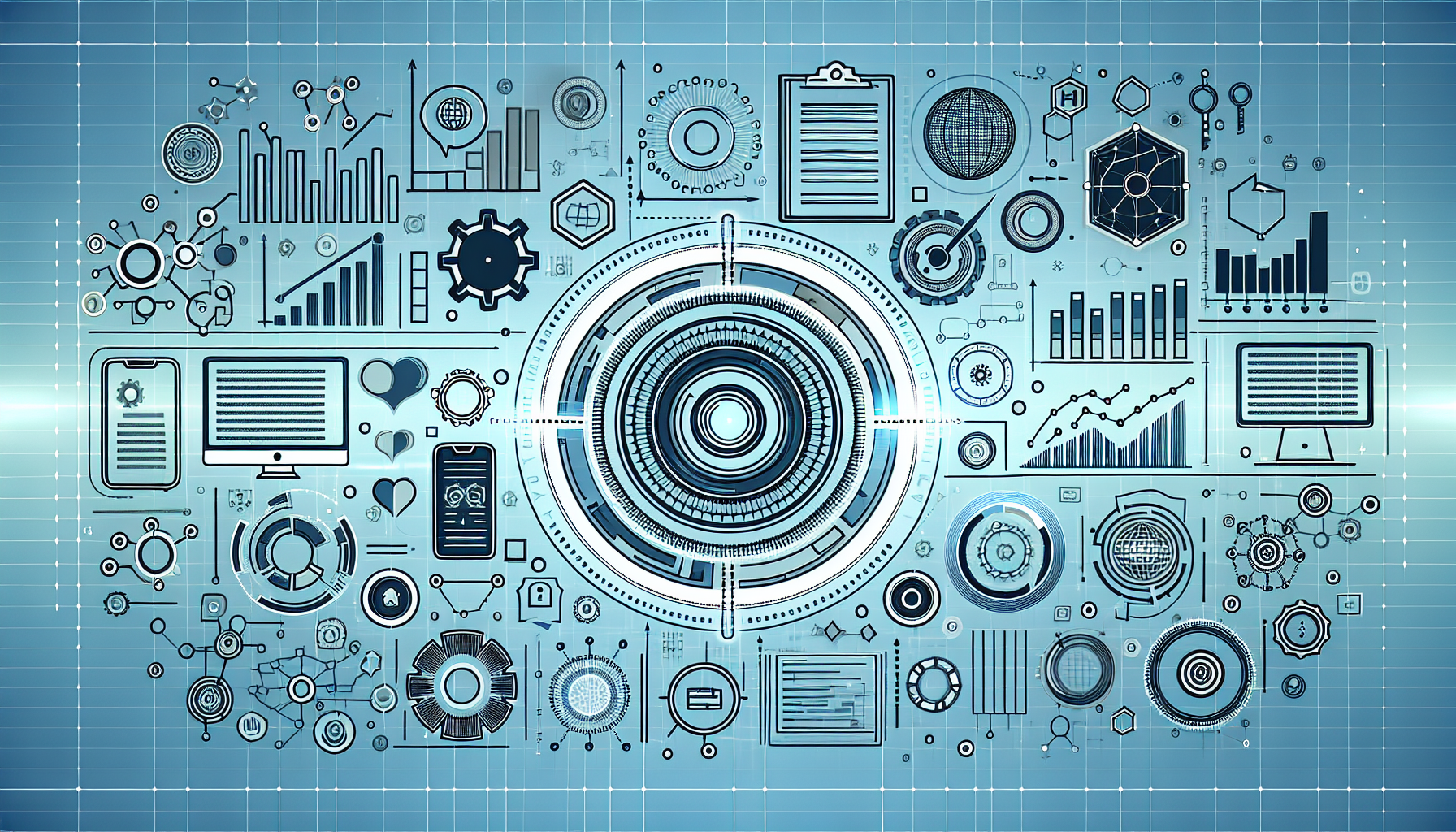
Data Visualization Tools
Data visualization makes it easier to understand complex data and presents analysis results more effectively. Here are some commonly used data visualization tools:
- Tableau: It stands out with its user-friendly interface and powerful visualization capabilities.
- Power BI: This tool, developed by Microsoft, is widely used especially in business intelligence applications.
- Matplotlib: This Python-based library is frequently preferred by data scientists.
- ggplot2: A powerful visualization library developed for the R programming language.
These tools help present data in a more understandable and effective way.
Machine Learning and Data Mining
Machine learning and data mining are advanced techniques used to extract meaningful information from large data sets. These techniques offer the ability to automatically learn and make predictions from data.
- Supervised Learning: The learning process done with labeled data. Examples include classification and regression algorithms.
- Unsupervised Learning: The learning process done with unlabeled data. Examples include clustering and dimensionality reduction algorithms.
- Deep Learning: Learning complex data using multi-layered artificial neural networks.
- Recommender Systems: Making recommendations based on users’ past behavior.
These techniques help optimize business processes by extracting valuable information from data.
Software and A tools
The software and tools used in data analysis speed up the analysis process and make it more effective. Here are some commonly used software:
- R: A powerful programming language for statistical analysis and data visualization.
- Python: Provides extensive library support for data science and machine learning.
- SAS: A powerful analysis software for working with large data sets.
- SPSS: Widely used for social sciences and market research.
- Excel: Widely used for small and medium-scale data analysis.
These software make data analysis processes more efficient and effective.
Frequently Asked Questions
1. What are the data collection methods?
Data collection methods include surveys, observation, experiments, and existing records.
2. Why is data cleaning important?
Data cleaning increases the quality of data and ensures that the analysis results are reliable.
3. What are descriptive statistics?
Descriptive statistics include mean, median, mode, standard deviation, and quartiles.
4. What data visualization tools can be used?
Data visualization tools include Tableau, Power BI, Matplotlib, and ggplot2.
5. What is the difference between machine learning and data mining?
Machine learning is the process of automatically learning and making predictions from data. Data mining aims to extract meaningful information from large data sets.
6. Which software can be used in data analysis?
Commonly used software in data analysis include R, Python, SAS, SPSS and Excel.